St Vincent’s Health – Automated Statement Reconciliation
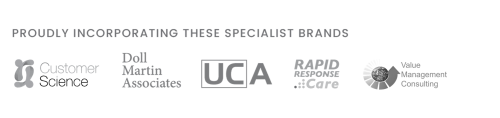
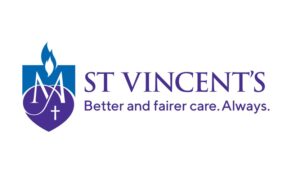
Project
Background
St Vincent’s Health Australia (SVHA) manages a network of 2 public hospitals, 10 private hospitals and 24 aged care communities across the country. To ensure financial accuracy and mitigate risks such as overpayments, missed invoices, and fraud, the Accounts Payable (AP) team must reconcile monthly supplier statements with the organisation’s ERP system (Microsoft D365). However, manual reconciliation processes constrained the team’s capacity and posed significant operational risks.
Problem Statement
With around 1,200 active suppliers, the SVHA Finance team could only reconcile statements from the top 50 suppliers each month for each division (Private Hospitals, Public Hospitals and Aged Care) due to resource limitations. This manual process consumed around 150 hours of the AP team’s time each month. Statements from other suppliers were reconciled only when capacity allowed, leaving the organisation vulnerable to errors and risks, including incorrect payments and inaccurate financial reporting.
Key challenges included:
- Manual reconciliation coverage was limited to 5-10% of suppliers monthly.
- Priority was given to suppliers with the highest spend, as they posed the greatest risk and were most critical. However, this approach left medium and small suppliers unreconciled, exposing them to potential cashflow challenges due to the risk of late payments.
- Significant time spent on repetitive, low-value tasks.
- Substantial business risks due to incomplete reconciliation.
Solution
SVHA partnered with Customer Science to deploy an intelligent document processing solution powered by UiPath Document Understanding. The approach combined machine learning (ML) and Generative AI (GenAI) to optimise reconciliation workflows. Key features included:
- Specialised ML Model: A custom-trained ML model identified and extracted data from supplier statements.
- Human-in-the-Loop: When the ML model lacked confidence, it flagged statements for review by the AP team, ensuring accuracy without full automation dependency. Any corrections are fed back into the ML model so that it improves over time.
- Generative AI Validation: A GenAI feature acts as a “second opinion,” cross-validating the ML model’s extractions. When both models agreed, statements were processed automatically, significantly reducing the need for manual intervention.
The automation integrated seamlessly with Microsoft D365, enabling the software robot to reconcile extracted data, flag discrepancies, and produce a detailed report of reconciliations and exceptions.
Results
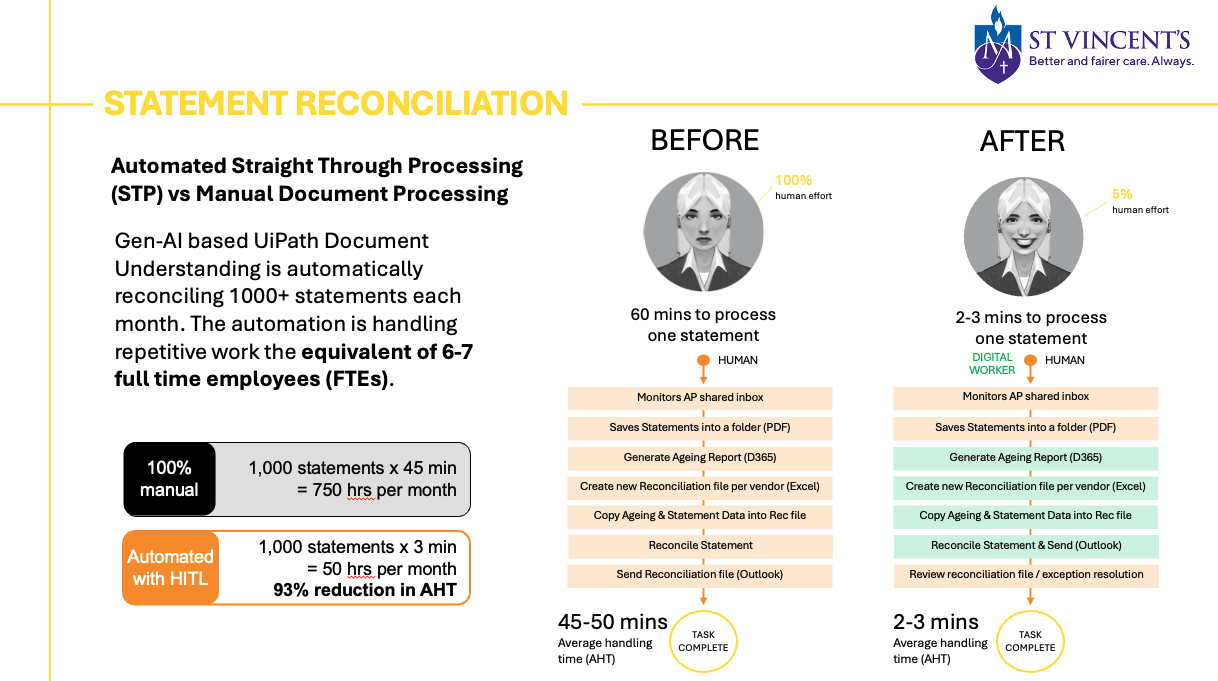
Benefits
The automation delivered transformative results:
- Increased Scope: Reconciliation coverage expanded from 5-10% to over 90%, reducing the risk of errors, overpayments, and fraud.
- Time Savings: Average case processing time dropped from 45 mins to 3 minutes. Even with a 90%+ increase in reconciliation coverage, the process improvement reclaimed 1200+ hours annually (~1 FTE equivalent) for the finance team, allowing them to focus on higher-value activities.
- Capacity uplift: The automation handles a workload equivalent to that of 6–7 full-time employees (FTEs).
- Efficiency Gains: Processing time was reduced by 93%+, enabling faster and more accurate financial reporting.
- Cost Efficiencies: The automation optimised resource allocation, reducing reliance on manual labour for tedious tasks.
- Enhanced Fraud Detection and Prevention: With a larger reconciliation scope and automated exception handling, the system provides improved oversight, making it easier to detect discrepancies, duplicate invoices, or fraudulent activity that might have gone unnoticed in manual processes. This strengthens financial controls and reduces the risk of financial mismanagement.
By automating this highly repetitive and labour-intensive task, SVHA enhanced financial accuracy, reduced operational risk, and empowered their team to focus on higher value work.